Publications
See Google Scholar for an up-to-date list of works.
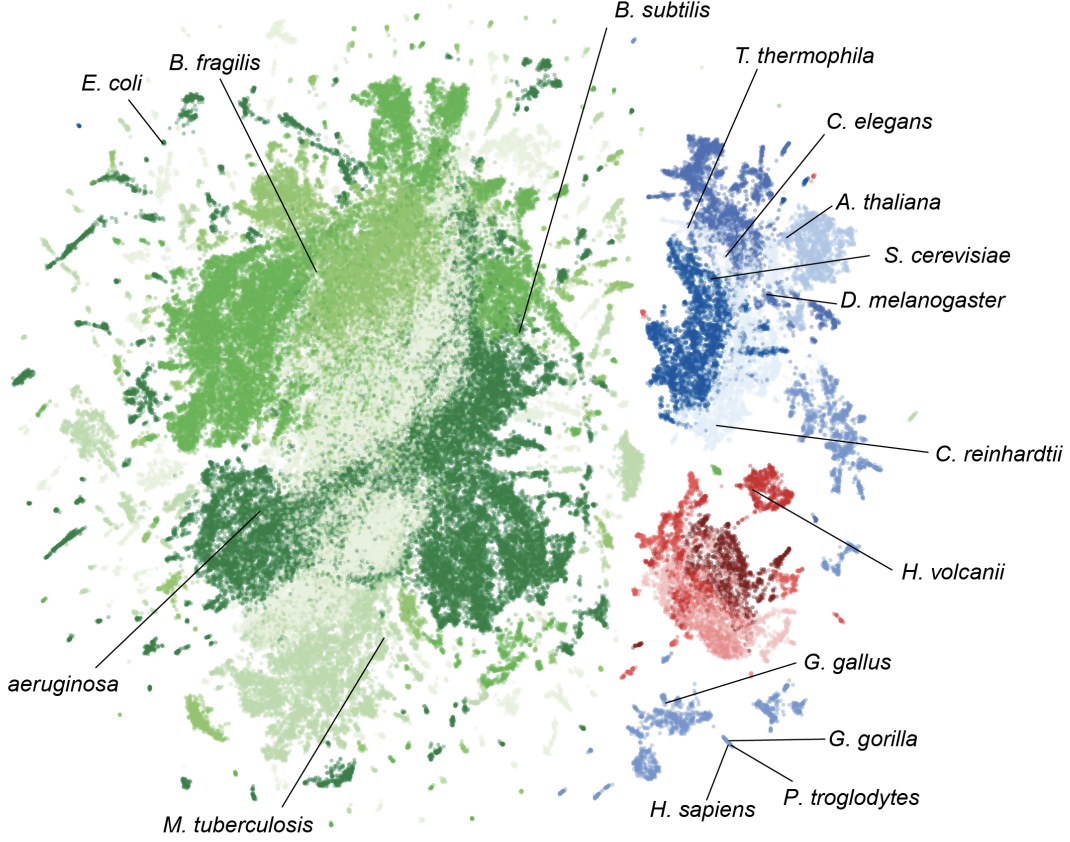
Genome modeling and design across all domains of life with Evo 2
bioRxiv, 2025
Evo 2 is a 40B parameter genomic foundation model capable of predicting functional impacts of genetic variations, autonomously learning biological features, and generating novel genomic sequences across all domains of life.
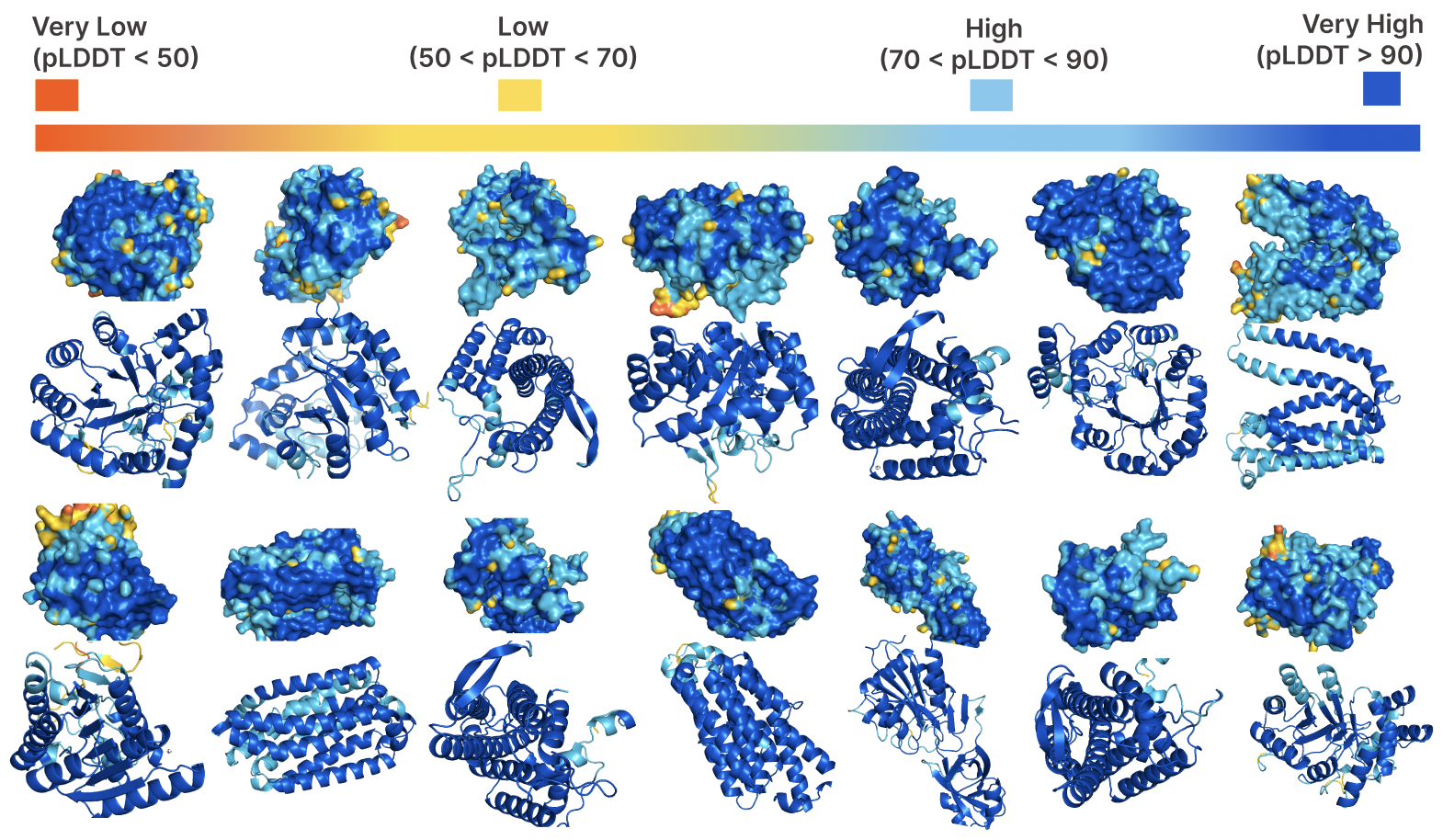
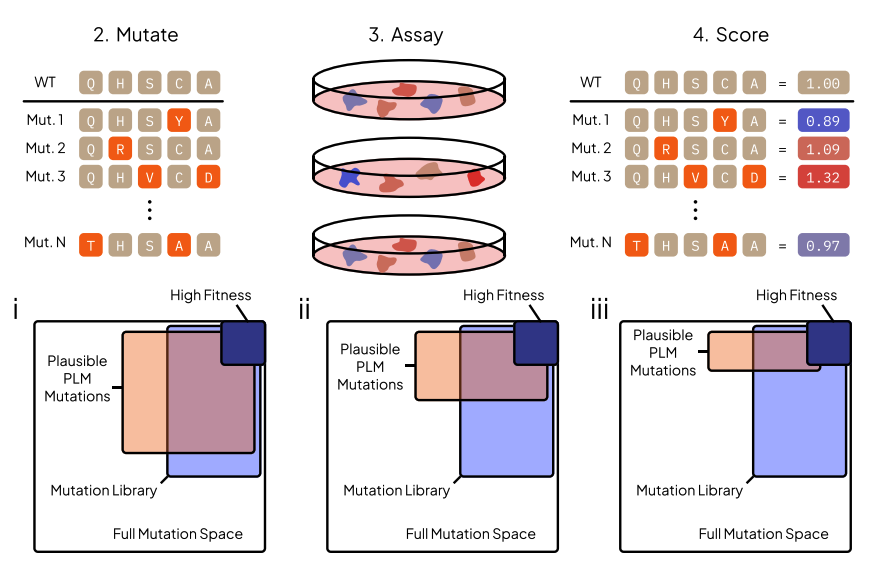
Protein Language Model Fitness Is a Matter of Preference
International Conference on Learning Representations (ICLR), 2025
Enabled by a one-pass pseudolikelihood algorithm, we find that pLMs capture artifacts of training data selection rather than true fitness landscape via influence functions.
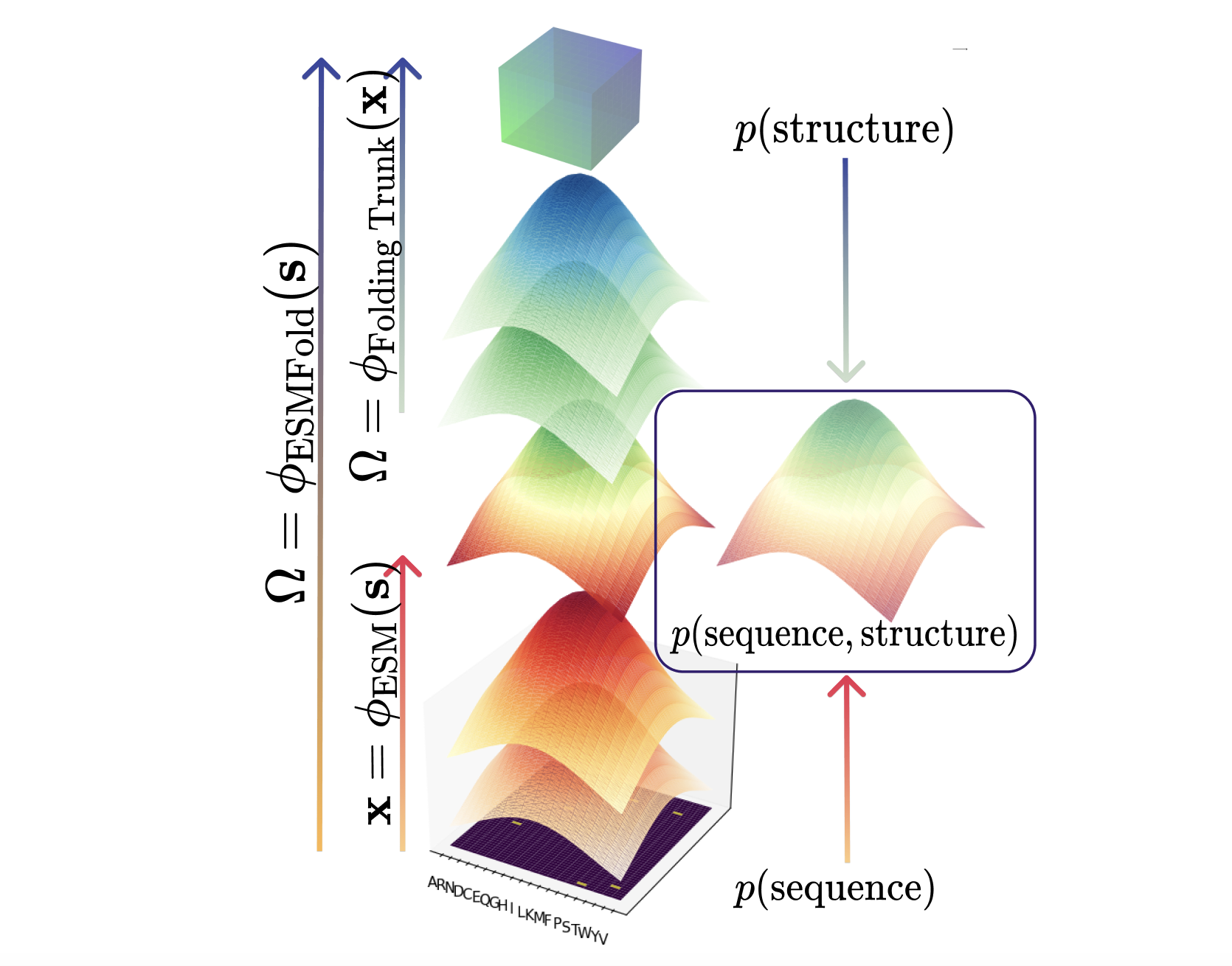
Tokenized and Continuous Embedding Compressions of Protein Sequence and Structure
bioRxiv, 2024
CHEAP is a joint embedding of protein sequence and structure that can be obtained from sequence alone, and unveil insights into the compressibilitiy, tokenizability, and mechanistic interpretability of protein folding models.
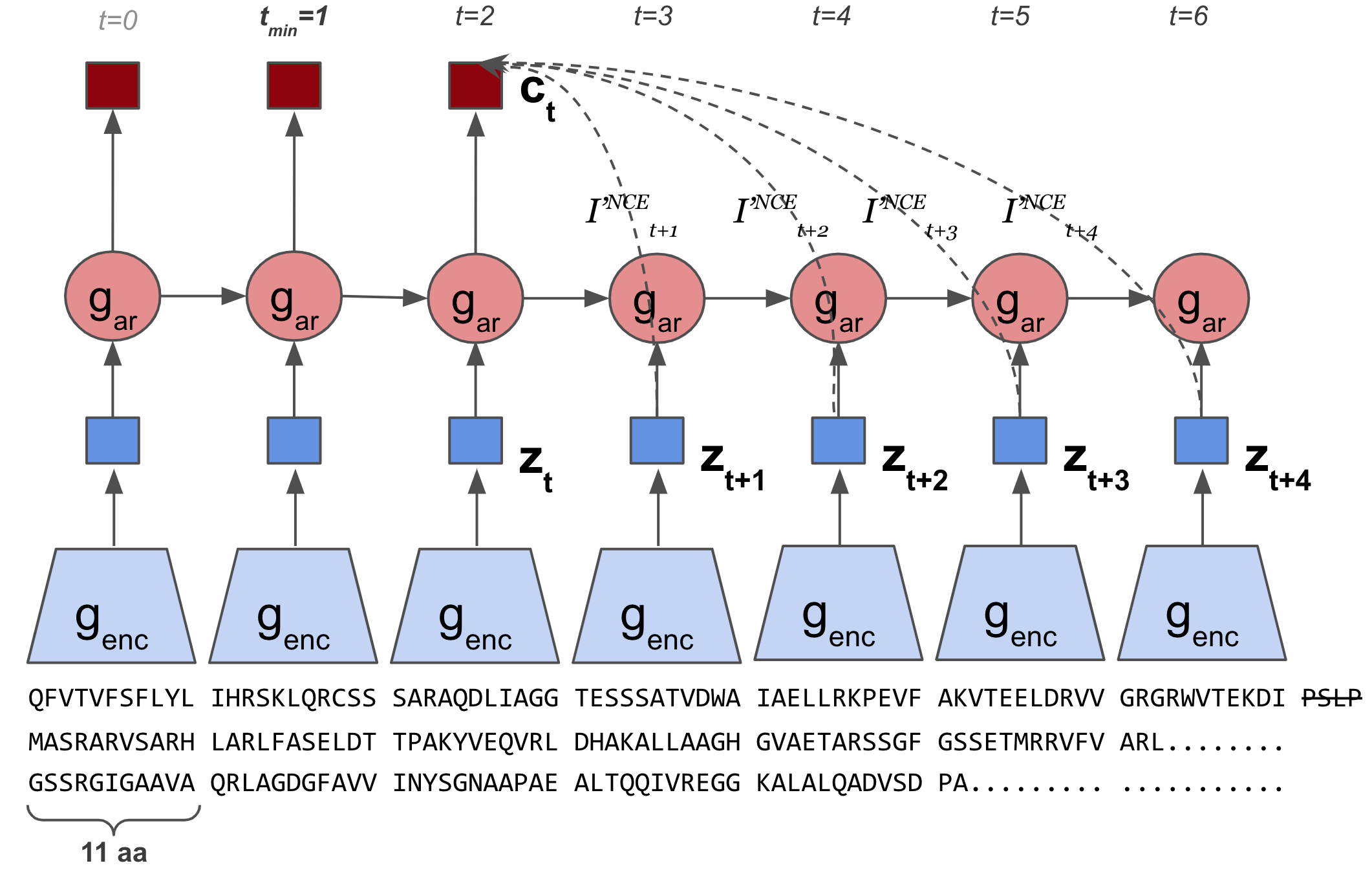
Self-Supervised Contrastive Learning of Protein Representations by Mutual Information Maximization
Machine Learning for Computational Biology (MLCB), 2020
CPCProt uses contrastive learning to learn a parameter-efficient way of embedding proteins, and performs competitively with large language models.
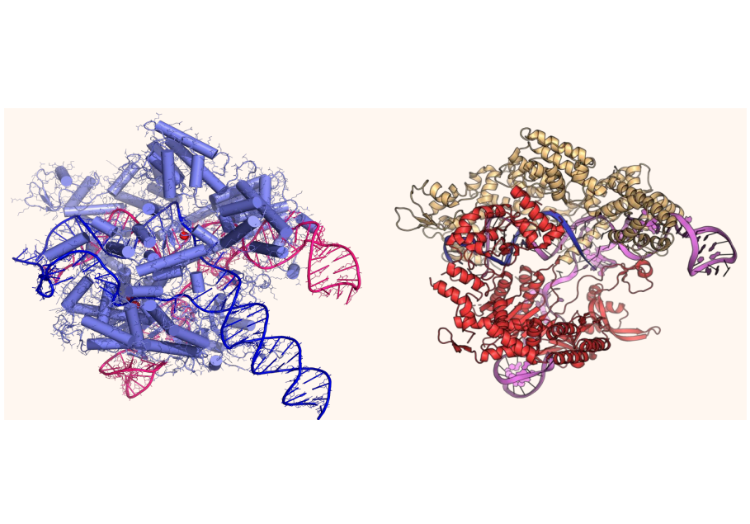
TOPH: Adapting A Contrastive Question-Answering Framework for Protein Search
ICML Workshop on Computational Biology, 2023
We present a protein semantic similarity search method for RNA-Guided endonuclease discovery, inspired by dense retrieval methods in open-domain question answering, and introduce a new dataset of CRISPR-Cas and evolutionary-related nucleases.
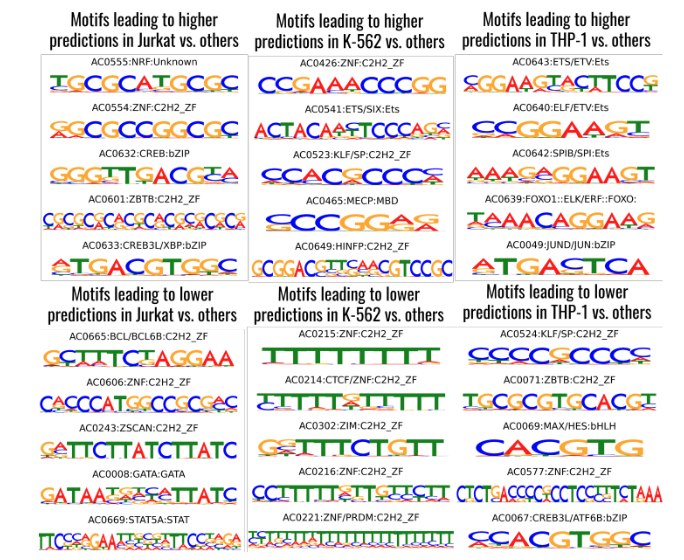
Pretraining strategies for effective promoter-driven gene expression prediction
bioRxiv, 2023
Pretraining and transfer learning strategies for improving model-based design of promoters for cell type-specific expression.
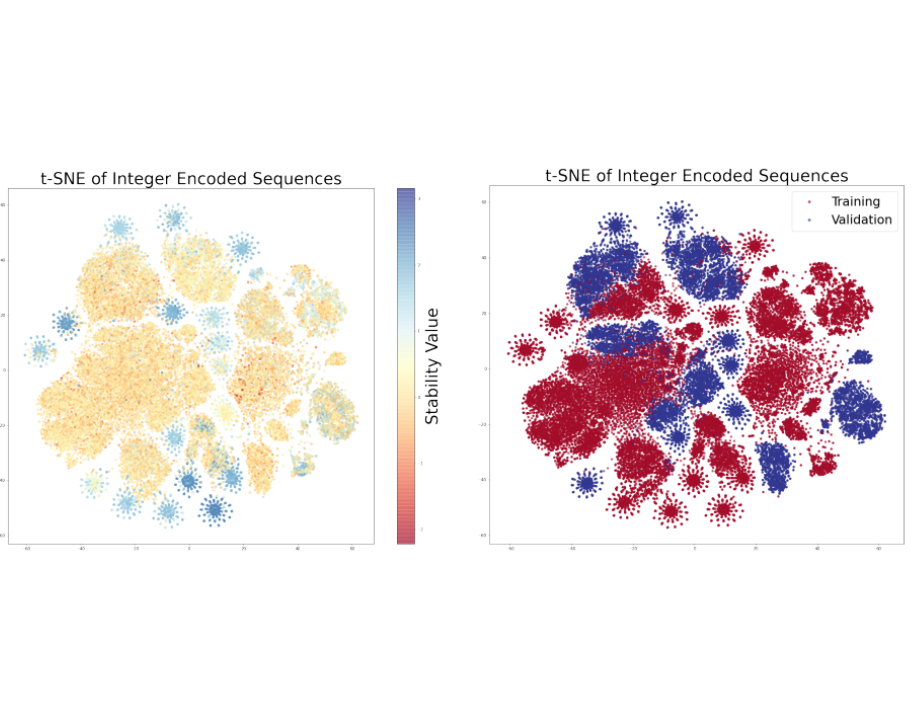
Data-Driven Optimization for Protein Design: Workflows, Algorithms and Metrics
ICLR Workshop on Machine Learning for Drug Discovery (MLDD), 2022
Strategies for data curation, model-training, optimization, and evaluation heuristics for data-driven proposals of novel de novo proteins.
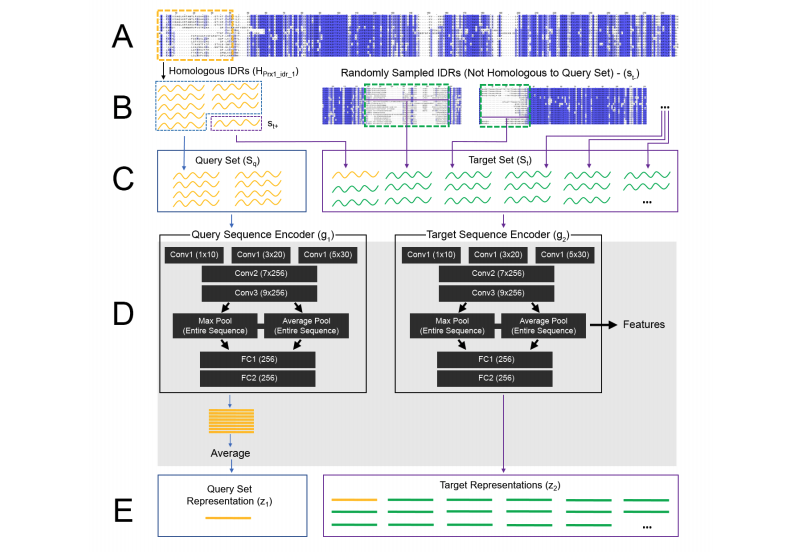
Discovering molecular features of intrinsically disordered regions by using evolution for contrastive learning
PLOS Computational Biology, 2022
Reverse Homology is a self-supervised method which captures evolutionary information by contrastive learning to discover molecular features of intrinsically disordered regions.
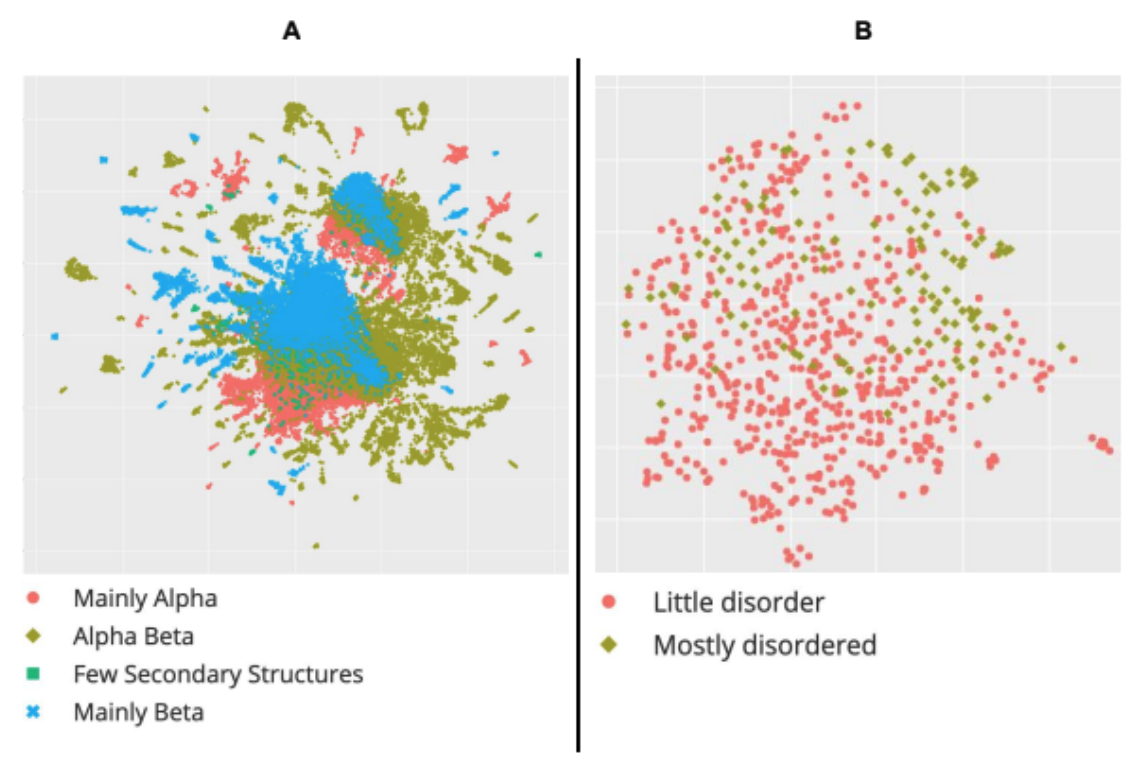
Learned embeddings from deep learning to visualize and predict protein sets
Current Protocols, 2021
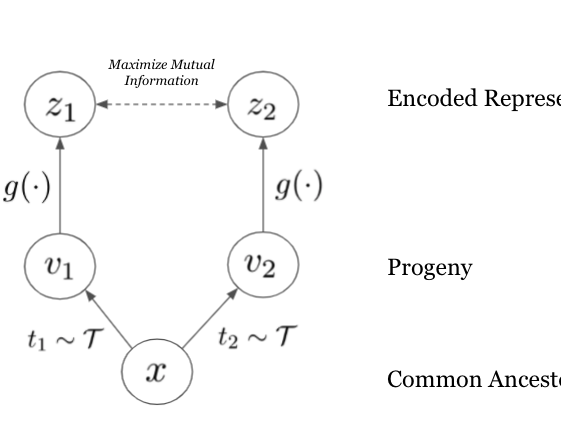
Evolution Is All You Need: Phylogenetic Augmentation for Contrastive Learning
Machine Learning for Computational Biology (MLCB), 2020
We outline how viewing evolution as natural sequence augmentation for contrastive learning recapitulates comparative genomics, and maximizes the mutual information between sequence and function.
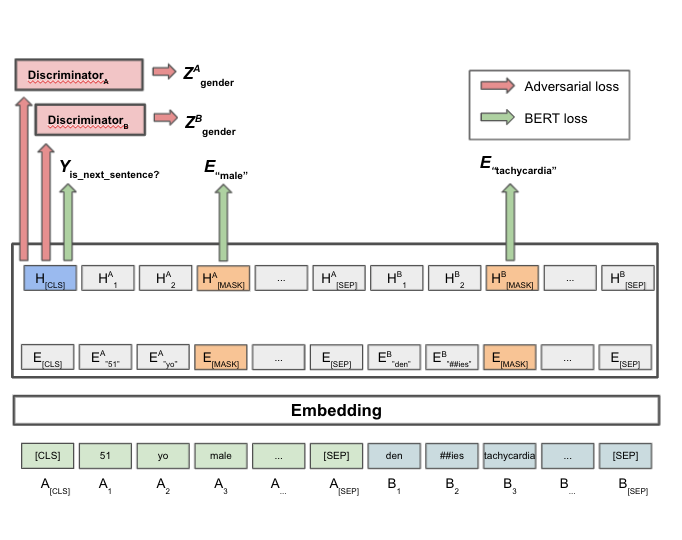
Hurtful Words: Quantifying Biases in Clinical Contextual Word Embeddings
ACM Conference on Health, Inference, and Learning (CHIL), 2020
We apply fairness definitions to quantify the cross-group bias in BERT embeddings pretrained on medical notes, and find statistically significant differences in classifier performance.
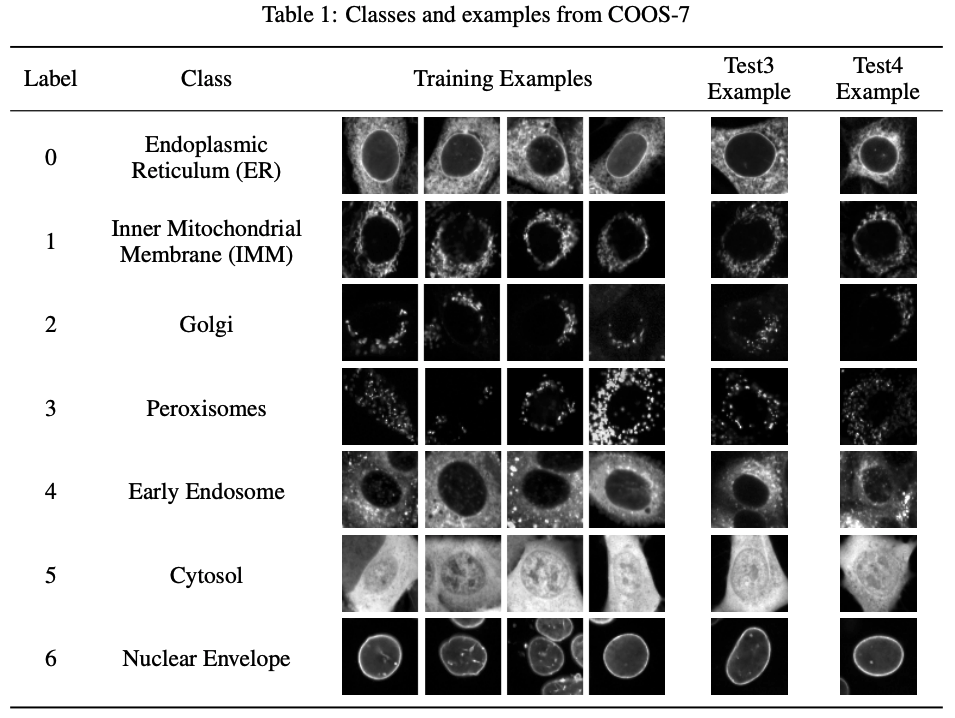
The Cells Out of Sample (COOS) dataset and benchmarks for measuring out-of-sample generalization of image classifiers
Neural Information Processing Systems (NeurIPS), 2019
Introduces the COOS-7 dataset to benchmark and evaluate the capacity of feature learning methods to generalize to natural distribution shifts in microscopy images.